Data mining and machine learning in retail business: developing efficiencies for better customer retention

The problem of customer acquisition and retention has been well studied. Number of soft techniques has been discussed earlier towards the development of retail marketing. However, the methods suffer to achieve higher performance and require some strategically approach. The application of data mining techniques has great impact in the development of retail marketing. Also, the volume of customer logs is higher which challenges the methods in identifying user interest in exact way and such issue can be handled with the inclusion of machine learning techniques. Towards this, a novel customer interest prediction algorithm with Multi Variant K-means clustering and pattern mining techniques is presented in this article. The method is focused to support E-Commerce systems. The method first identifies the purchase histories and enquires of various users. The logs have been clustered using Multi variant k-means clustering algorithm. Second, the method identifies the list of purchase patterns. From the patterns being generated, the method identifies the concrete interest of the user and identifies the similar interested users. Using this, set of recommendations has been generated for the user which has been used to populate advertisements, placing banners in user web page, and so on. By identifying the user interest according to their purchase pattern and by generating the recommendations based on the logs of similar interested users, the method supports the customer retention in higher ratio.
This is a preview of subscription content, log in via an institution to check access.
Access this article
Subscribe and save
Springer+ Basic
€32.70 /Month
- Get 10 units per month
- Download Article/Chapter or eBook
- 1 Unit = 1 Article or 1 Chapter
- Cancel anytime
Buy Now
Price includes VAT (France)
Instant access to the full article PDF.
Rent this article via DeepDyve
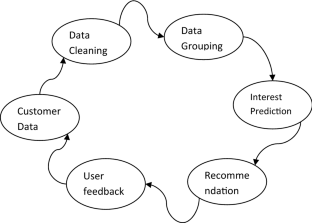
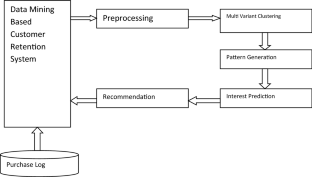
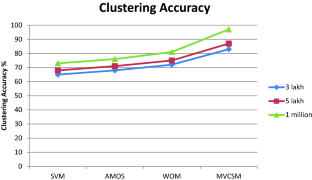
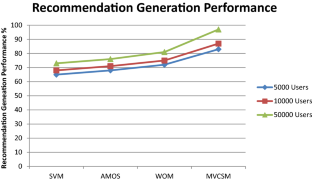
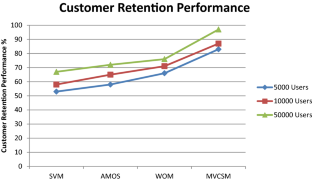
Similar content being viewed by others
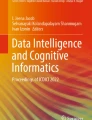
Customer Analytics Research: Utilizing Unsupervised Machine Learning Techniques
Chapter © 2023
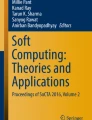
Comparative Analysis of Clustering Techniques for Customer Behaviour
Chapter © 2018
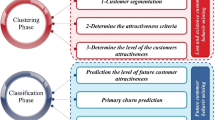
Customer Behavior Mining Framework (CBMF) using clustering and classification techniques
Article Open access 11 August 2018
Explore related subjects
References
- Adhiambo C, Odhiambo M (2012) Social media as a tool of marketing and creating brand awareness, Business Economics and Tourism
- Ahmeda RAE-D, Shehaba ME, Morsya S, Mekawiea N (2015) Performance study of classification algorithms for consumer online shoppingattitudes and behavior using data mining. In: 5th International conference on communication systems and network technologies.
- Algharbat RS (2020) Social commerce in emerging markets and its impact on online community engagement Information System Frontiers. Springer, Berlin Google Scholar
- Asian News Service (2012) India’s retail industry to grow to $1.3 trillion by 2020: FICCI—Yahoo! News India. http://in.news.yahoo.com/indias-retail-industry-grow-1-3-trillion-2020-121004703--finance.html. Accessed 1 Jan 2013
- Baskar M, Gnansekaran T (2016) Developing efficient intrusion tracking system using region based traffic impact measure towards the denial of service attack mitigation. J Comput Theor Nanoscience 14:3576–3582 ((ISSN: 1546-1955 (Print): EISSN: 1546-1963 (Online))) ArticleGoogle Scholar
- Baskar M, Ramkumar J, Rathore R, Kabra R (2020) A deep learning based approach for automatic detection of bike riders with no helmet and number plate recognition. Int J Adv Sci Technol 29(4):1844–1854 ((ISSN: 2005-4238)) Google Scholar
- Cox DD (2017) Social media marketing and communications strategies for school superintendents. JEA 52(6)
- Crittenden V (2015a) Digital and Social Media Marketing in Business Education. JME 37(2):131–132 Google Scholar
- Crittenden VL (2015b) Digital and social media marketing in business education: implications for the marketing curriculum. JME 37(2):71–75 Google Scholar
- Foux G (2006) Consumer-generated media: get your customers involved. Brand Strategy 38–39
- Frederiksen LW (2015) 3 Key digital marketing skills students don’t learn in college. Retrieved from http://www.fastcompany.com/welcome.html?destination=http://www.fastcompany.com/3041253/3-key-digital-marketing-skills-students-dont-learn-in-college
- Gupta S (2012) A Billion+ Customers need a million sales and service touch points. FICCI blog. http://blog.ficci.com/retail-india/1174/. Accessed 1 Jan 2013
- Jobber D (2007) Principle and practice of marketing, McGraw Hill Education
- Ju C, Guo F (2008) Research and application of customer churn analysis in retail chain industry. In: IEEE, International symposium on electronic commerce and security, 2008
- Kaplan AM, Haenlein M (2010) Users of the world, unite! The challenges and opportunities of social media. Bus Horiz 53(1):59–68 ArticleGoogle Scholar
- Livinus UT, Chelouah R, Senoussi H (2016) Recommender system in big data environment. Int J Comput Sci Issues 13(5):1–10 ((ISSN (Print): 1694-0814 | ISSN (Online): 1694-0784)) ArticleGoogle Scholar
- Lucintel (2012) Global retail industry 2012–2017: trend, profit, and forecast analysis. Market research reports. Lucintel, Dallas Google Scholar
- Mangold WG (2009) Social media: the new hybrid element of the promotion mix, vol 52, issue 4. Elsevier, Business Horizons
- Mukhaini E (2014) The adoption of social networking in education: a study of the use of social networks by higher education students in Oman. JIER 10:143–154 ArticleGoogle Scholar
- Nair M (2011) Understanding and measuring the value of social media. J Corp Acc Finance
- Piramuthu S, Farahani P, Grunow M (2013) RFID-generated traceability for contaminated product recall in perishable food supply networks. Eur J Oper Res 225:253–262 ArticleMathSciNetGoogle Scholar
- Rita P (2019) The impact of e-service quality and customer satisfaction on customer behavior in online shopping. Heliyon 5(10):e02690 ArticleGoogle Scholar
- Srinivasan K, Saravanan S (2015) A study on financial constraints and challenges in retailing trade: a bird’s eye view. Adv Manag 6(1):21–25 Google Scholar
- Stephen AT (2015) The role of digital and social media marketing in consumer behavior. Curr Opin Psychol 10:17–21 ArticleGoogle Scholar
- Suchithra M, Baskar M, Ramkumar J, Kalyanasundaram P, Amutha B (2020) Invariant packet feature with network conditions for efficient low rate attack detection in multimedia networks for improved QoS. J Ambient Intell Human Comput. https://doi.org/10.1007/s12652-020-02056-1ArticleGoogle Scholar
- Winer RS (2009) New communication approaches in marketing: issues and research directions. J Interact Mark 23:108–117 ArticleGoogle Scholar
Acknowledgements
The authors want to acknowledge the help of all the people who influenced for their reasonable comments.